Avoiding risk bias through effective building data practices
Here's an extreme hypothetical that demonstrates how risk bias can negatively impact policyholders — and a solution.
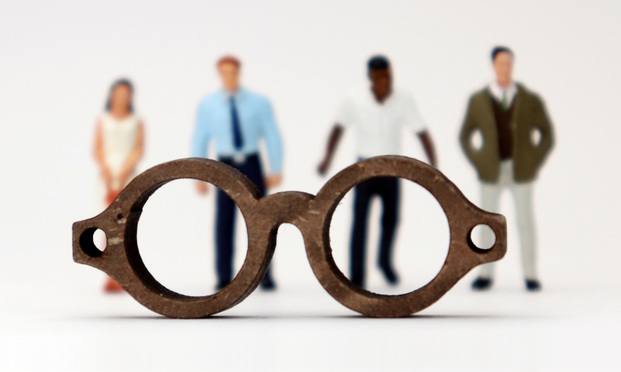
Bias is defined as, “prejudice in favor of or against one thing, person, or group compared with another, usually in a way considered to be unfair.”
Typically, we understand biases through the lens of our social constructs.
But in the context of risk management, risk bias can be quite an interesting phenomenon as humans are incapable of solving it independently.
Let’s use an extreme hypothetical to demonstrate how risk bias could impact the likelihood of compounded losses on a particular property or portfolio.
Meet Greg…
Greg is a risk management professional tasked with properly accounting for and implementing a risk management plan for all of the properties owned by Firm X across the continental United States. Firm X’s executives have cleared Greg to pursue a policy that can cover the full spectrum of loss scenarios across their portfolio of warehouse facilities.
Greg starts with what he’s most familiar with. He grew up in the wine country of Northern California and can remember his family evacuating twice. Greg follows the difficulty of securing commercial coverage in that region. So he starts his work there, moving west to east across the company portfolio. Greg accounts for all loss histories across the portfolio and successfully acquires syndicated coverage across several commercial insurers.
Nine months later, two of Firm X’s facilities in Northern Michigan experienced a deep freeze that causes both buildings to have bursting pipes, making the facility unusable for two weeks and causing loss that was NOT covered in the policy he procured for those facilities. The native Northern Californian had good intentions, but his perspective was heavily influenced by his upbringing and his lack of understanding of North Eastern weather trends. Firm X had to repair the two facilities on their own and lost more than three weeks of business activity.
Certainly, this is an extreme example; but it is demonstrative of what risk bias is.
Solving risk bias through effective data practices
Fortunately, risk bias doesn’t have to be a self-fulfilling prophecy. In nearly every scenario in which a historical record, a document of record or comprehensive data sets are available, we can inoculate against our human tendency for skewed perceptions of reality.
Shana Lebowitz and Samantha Lee recently wrote an informative article published by Business Insider that explored “cognitive biases that screw up your decisions.” In the context of risk planning for commercial properties, we’ll focus on a handful of them and how data can help mitigate or eliminate the potential for bias.
No. 1: The clustering illusion is the tendency to see patterns in random events. Our fictional character, Greg, saw random wildfires in his upbringing and interpreted them to mean he needed to spend extra time planning for the possibility of wildfire. Though his intentions were pure, he may not have dedicated enough time or energy to understand the risks associated with specific locations that are not at all similar to where he was raised. Said differently, just because you’ve experienced losses in one particular geography, context or building doesn’t mean it will continue and won’t happen elsewhere. Centralized, organized, quality data helps us take an unbiased approach to manage risk.
No. 2: Confirmation bias is the tendency to listen to only information that confirms our perceptions. This can happen in risk management, especially at the executive level. Property managers may be alerting us to certain problems with their buildings, and we tend to pay attention to those we perceive as big problems and ignore the rest. Quality data helps us to focus on all important risks, not just those we selectively choose as important for one reason or another.
When quality data is organized and sorted by TIV (Total Insurable Value), year built, or other variables with evidentiary documents at your fingertips, perception is replaced by reality to provide a more complete picture.
No. 3: The ostrich effect, or burying your head in the sand to avoid negative or dangerous information, is easier to avoid when information is presented as quality data instead of rough assumptions or plain conjecture. Unencumbered with our built-in biases, data helps break through to those needing to make decisions based on the information.
No. 4: The recency effect, or the tendency to overweigh what’s happened most recently, can greatly skew our perception of risks. If Hurricane Ian and the fallout from the storm are at the forefront of our minds, we might lend an extra focus for planning around storms and coastal flooding. But having instant access to loss data that tracks back years is, in most cases, much more useful than the most recent devastating event we’ve experienced.
Linking the losses back to the property over multiple years uncovers important trends, which can help balance proactive versus reactive expenditures on hazard-prone properties.
No. 5: Blind spot bias, or the inability to recognize your own biases and overcome them, is demonstrated by our Greg. Risk managers have long careers in highly specialized professions. When risk managers resolve to approach things in the same manner as they have in the past, it leads to the near certainty that blind spots will develop. Accurate and accessible data can defend against this inevitably.
Businesses increasingly rely on a technology stack of CRM, marketing, and HR tools to help run their businesses. Today’s risk management professionals have more and more tools available. Embracing such solutions can ensure success.
Erroin Martin (erroin@onarchipelago.com) is the chief revenue officer at Archipelago, an AI-driven technology platform that connects and transforms property risk data for large commercial property owners to deliver optimal insurance and business outcomes.
See also: